*This post is based on a talk given by Bob Kasenchak at the 2023 Information Architecture Conference. It was a detailed 20-minute presentation designed for IA practitioners. Our goal with this post, however, is to distill it down to its foundational thesis and invite a discussion about the important differentiation between Subject and Topic Taxonomies. Why? Let’s explore is-ness and i-verse-ness.
First, we have a central conflict in taxonomy: how to organize information that accurately represents your enterprise’s world – domain, business context, etc. – and simultaneously organize the same information so your target audiences can easily find it. In short: categorizing things according to what they are is sometimes in conflict with where people will find them.
That is to say: your navigation taxonomy is not a classification system.
Imagine being asked to construct a taxonomy for tagging web pages, listing products, and categorizing documents, images and other assets. (NOTE: We’re excluding taxonomies used specifically for navigation as they follow their own logic.) And imagine for this project, as it’s easy to do, that we think of and use Subject and Topic taxonomies interchangeably. We will quickly create a problem at the very foundation of our Information Architecture project.
What do we mean?
Subject: Dogs means a list of breeds (and perhaps including examples of specific dogs).
Topic: Dogs includes stuff related to dogs. All the stuff in the dog-i-verse.
Subjects are specific kinds of things.
Topics are stuff that reminds me of, or is associated with things (or that a user might need to find quickly, for example).
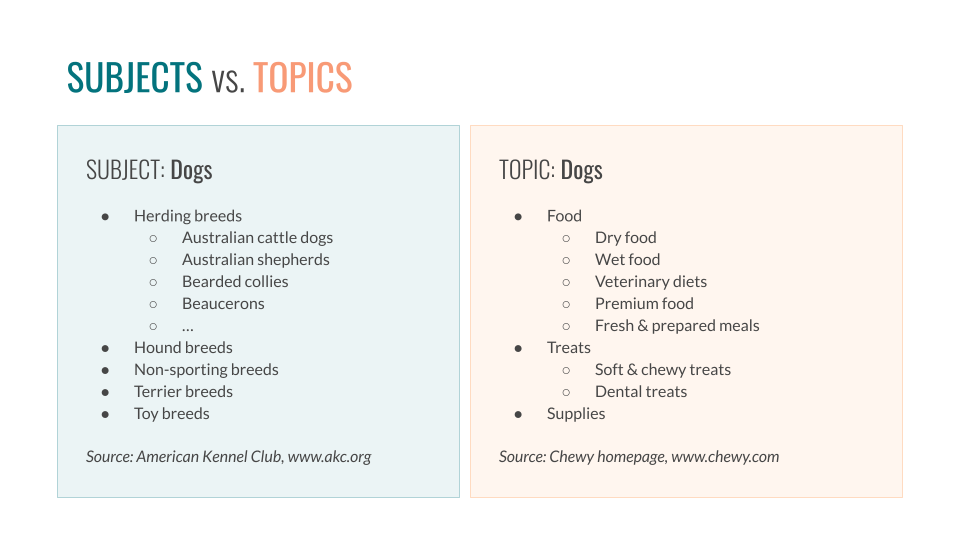
Subject Taxonomies and Topic Taxonomies are structured similarly. They both feature hierarchically arranged trees of words describing concepts. The difference between Subject and Topic Taxonomies is the criteria by which we assign hierarchical relationships. In short: some things are dogs, and some are not (but are in the dog-i-verse).
Subject taxonomies are arranged according to is-ness.
Topic taxonomies are groups of subjects related by a common theme – the -i-verse-ness.
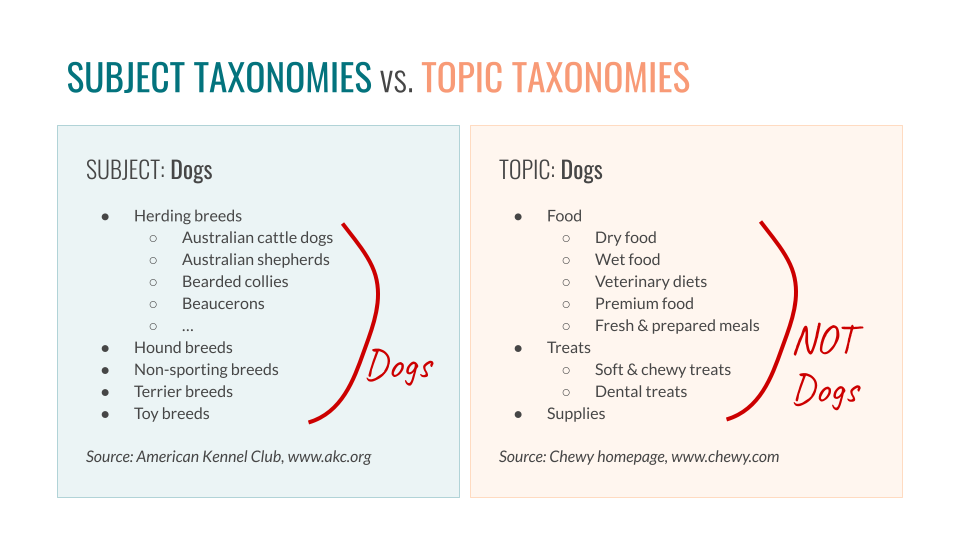
To provide another example: Skiing is a Subject. It includes sub-Subjects like Downhill, Slalom, and Cross-Country Skiing. Is-ness. However: Skis are not Skiing. Ski resorts, ski equipment, and skiers are not Skiing. They all properly belong to the Topic of Skiing. I-verse-ness. Is-ness vs i-verse-ness: We can do this with any Subject and any Topic. (And we should.)
Many taxonomies mix topics and subjects (and there are cases where this is necessary). However, it should be avoided in most/all instances where topics and subjects are mindlessly interchanged as one and the same. As soon as we give ourselves license to mix Topics and Subjects we open ourselves up to Taxonomy Problems, like asserting that Purina Dog Chow is the same Kind of Thing as a Poodle (which is obviously an error).
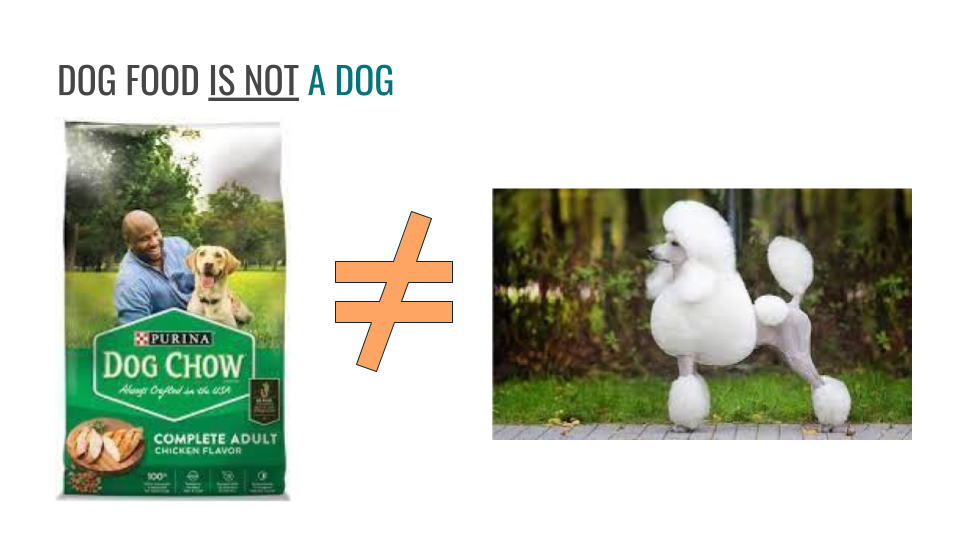
And this is where we pave the way to the Machine Learning team to break down the door in search of your Taxonomy (insert action movie score here).
Machine Learning Is Coming for Your Taxonomies and Metadata
If they haven’t already, soon somewhere, someone is going to suggest, “Hey, can’t we just do this Information Architecture thing with ML?” And the Machine Learning folks will see your taxonomy and structured metadata as a way to bootstrap their ML routines.
The error here is that Subject Taxonomies are indeed GREAT for inference. Topic taxonomies are…not. Because, as we have seen, they imply class relationships that are false.
Consider a common search on a major ecommerce retailer (in this case, Crate & Barrel – note that this is just an example, not a problem with their search!) for coffee:
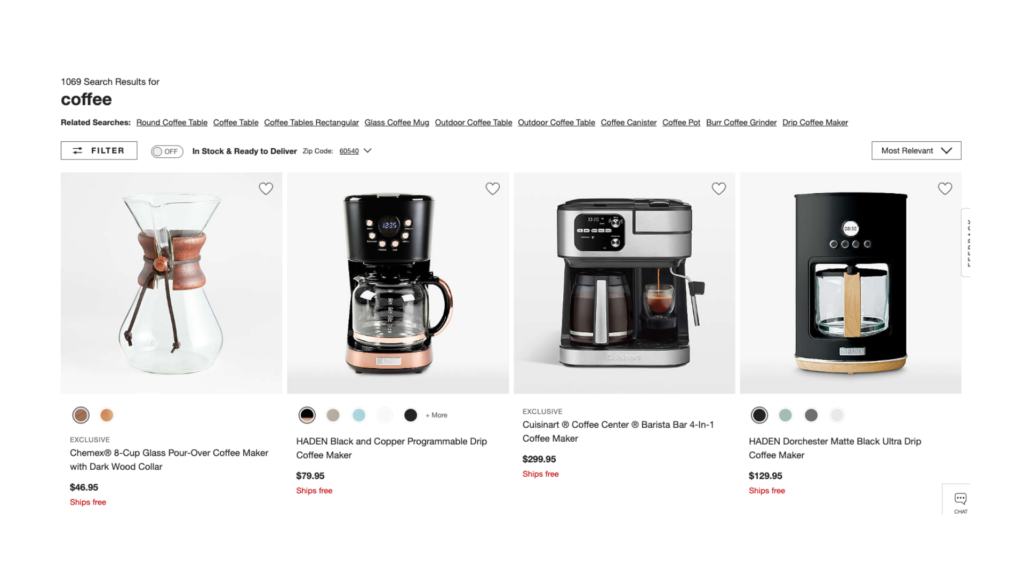
(You can perform the same search yourself and scroll down to see more.) Naturally (as they don’t actually sell coffee) the results are for coffee makerscoffeemakers, coffee accessories, coffeeware, and other things in the coffee-i-verse. Which is great! People should be able to find things this way. The point, though, is that we don’t want to use this to train a ML model: none of these things are coffee.
Can’t We All Just Get Along? Yes.
So we want to create good, structured data for ingestion by ML applications. This doesn’t need to be a turf battle between the IA Silo and the AI Silo for total and final control of how we model and understand things in our organizations. Our collective goal, afterall, is to create the most intuitive, fluid, and aligned connection between information and audience. We want to create elegant IA structures that organize things strictly (according to what they are) and also in a way useful to humans so they can find what they are looking for. We want to deliver is-ness and i-verse-ness to create connected-ness.
By harnessing the power of AI, and combining it with – let’s call it human-ness – we can create much better outcomes than either can alone. We have a vested interest in these harmonious outcomes, because one of the values that gives our work purpose is creating meaningful connections. We’re guessing that’s also an important principle for your enterprise.
Chances are this debate is starting to bubble up (if not full-on already raging) within your organization. We’d welcome the opportunity to learn about some of your challenges and dilemmas as you try to navigate this emerging era.